ALGORITHMS, AI, AND FERPA, OH MY
This newsletter is sent in partnership with the Data Integration Support Center (DISC) at WestEd.
This newsletter is sent in partnership with the Data Integration Support Center (DISC) at WestEd. DISC provides expert privacy, policy, and legal assistance for public agencies nationwide. For more information, check out DISC’s website.
NEWSLETTER: ALGORITHMS, AI, AND FERPA, OH MY
Over the past few months, there's been significant movement at the federal level that’s going to impact state-level integrated data systems. We’ve poured through the details so you don’t have to. Read on to learn more about the highlights.
A Markup investigation claims that Wisconsin’s Early Warning System generated “false alarms about Black and Hispanic students at a significantly greater rate than it does White students” and a major research study found the system to be inaccurate at the individual school level;
There is a lot of federal attention on AI right now, as seen in USEd’s recent report “Artificial Intelligence (AI) and the Future of Teaching and Learning: Insights and Recommendations”, a National AI R&D Strategic Plan, and a new Request for Information (RFI) on National Priorities for Artificial Intelligence;
The White House’s Fact Sheet on “Actions to Protect Youth Mental Health, Safety & Privacy Online” includes an announcement that USEd is planning to commence FERPA rulemaking;
The announcement was part of the Administration’s recently released Fact Sheet on New Actions to Promote Responsible AI Innovation that Protects Americans’ Rights and Safety.
New study claims ineffective algorithms used for Wisconsin’s early warning system and the Markup raises concerns about using “Race and Income to Label Students ‘High Risk.’”
Everyone should read the new investigation by the Markup, a nonprofit newsroom, and the just-posted study “Difficult Lessons on Social Prediction from Wisconsin Public Schools” by researchers based out of the University of California, Berkeley, using state-wide data on over 220,000 students between 2013 and 2021 that was provided by the Wisconsin Department of Public Instruction.
While these reports focused on Wisconsin, it’s important to remember that multiple states are using or planning to start using predictive algorithms in their early warning systems:
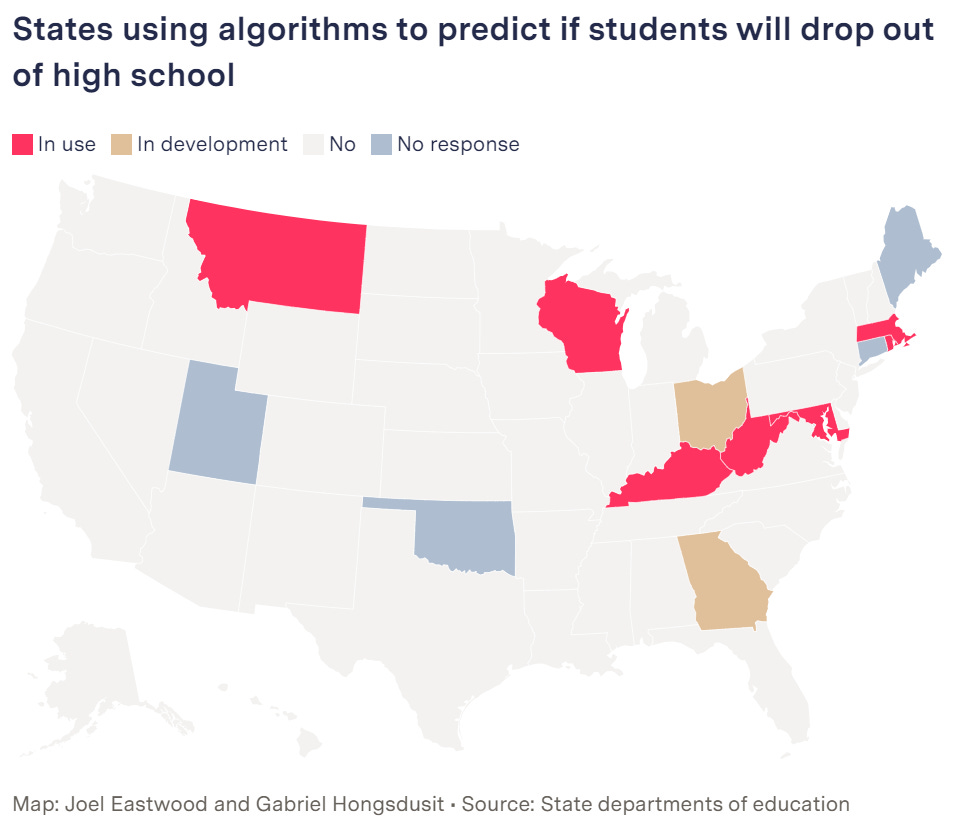
A quick caveat: The early warning system these reports focus on uses predictive algorithms. There are numerous early warning systems that do not use algorithms or machine learning to predict student outcomes.
Why this matters: The average stakeholder is unlikely to differentiate between early warning systems, statewide longitudinal data systems, integrated data systems, and other governmental data systems. This means that press coverage like this may be interpreted as representing multiple different types of data systems, regardless of differences in their purpose, implementation, or privacy protections.
These were some of the findings:
False Alarm: How Wisconsin Uses Race and Income to Label Students “High Risk”:
“Wisconsin uses a computer model to predict how likely middle school students are to graduate from high school on time. Twice a year, the Dropout Early Warning System [(DEWS)] predicts each student’s likelihood of graduating based on data such as test scores, disciplinary records, and race. But state records show the model is wrong nearly three quarters of the time it predicts a student won’t graduate. And it raises false alarms about Black and Hispanic students at a significantly greater rate than it does White students.”
“The algorithm’s false alarm rate—how frequently a student it predicted wouldn’t graduate on time actually did graduate on time—was 42 percentage points higher for Black students than White students, according to a DPI presentation summarizing the analysis…The false alarm rate was 18 percentage points higher for Hispanic students than White students. DPI has not told school officials who use DEWS about the findings nor does it appear to have altered the algorithms in the nearly two years since it concluded DEWS was unfair.”
“Students we interviewed were surprised to learn DEWS existed and told The Markup they were concerned that an algorithm was using their race to predict their future and label them high risk.”
“‘I wonder at the ways in which these risk categories push schools and districts to look at individuals instead of structural issues—saying this child needs these things, rather than the structural issues being the reason we’re seeing these risks,’” said Tolani Britton, a professor of education at UC Berkeley, who co-wrote the forthcoming study on DEWS. “‘I don’t think it’s a bad thing that students receive additional resources, but at the same time, creating algorithms that associate your race or ethnicity with your ability to complete high school seems like a dangerous path to go down.’”
“Difficult Lessons on Social Prediction from Wisconsin Public Schools”:
DEWS “is highly accurate when evaluated across the entire population of students in Wisconsin public schools…Hence, predictions are not much better within each school than randomly guessing which students are at risk of dropping out. Even if a particular school has effective educational interventions, administering them on the basis of DEWS scores is no better than a random allocation of the intervention.”
“Within the same school environment, graduation outcomes are almost entirely unrelated to the extensive set of individual student features, including race, gender, and test scores.”
“We estimate that assigning students into higher predicted risk categories improves their chances of graduation by less than 5%. Nevertheless, the 95% confidence interval for this estimated effect firmly includes zero.”
“Compared to investing in other community-based social service interventions, the decision to fund and implement sophisticated early warning systems without also devoting resources to interventions tackling structural barriers should be carefully evaluated in light of these school-level disparities.”
“Evidently, identifying a large subset of students that needs help is in many ways a trivial task. Not only can it be done without the use of individual student information, it can also be done without any machine learning at all. This school based strategy requires significantly less effort and technical sophistication than designing a well-functioning machine learning system. The relevant question here is not who needs help?, but rather it’s clear who needs help, what should we do about it?.”
There haven’t been many responses on the article and research so far, but I did enjoy reading “A Critique of The Markup’s Investigation into Predictive Models of Student Success”. It acknowledges in the first paragraph that DEWS “appears not to be fit for purpose and needs to be substantially improved” and discussed the importance of the language used to describe predictions:
“Additionally, it’s important to avoid self-fulfilling prophecies, where the language used suggests that nothing can be done to improve a student’s odds or outcome. It’s too easy to interpret phrases like “high risk” as being an essential and unchanging part of a student’s being, not something that can and will change over time, or that could be changed with effort. Ideally, the model should be identifying students who would benefit from additional support, and describing them as such.”
This is not the first time The Markup has investigated predictive algorithms in education: in 2021, they published “Major Universities Are Using Race as a “High Impact Predictor” of Student Success: Students, professors, and education experts worry that that’s pushing Black students in particular out of math and science.” Within a month, Texas A&M Drop[ped] “Race” from Student Risk Algorithm Following Markup Investigation”.
The Markup also recently reported that “It Takes a Small Miracle to Learn Basic Facts About Government Algorithms,” highlighting Wired’s March article “Inside the Suspicion Machine,” the result of an investigation “of the fraud-detection algorithm used by the city of Rotterdam, Netherlands, to deny tens of thousands of people welfare benefits.” Both The Markup and Wired flag that these systems are also actively used in the U.S.
State agencies and integrated data systems’ staff should keep a close eye on The Markup’s reporting, and consider taking advantage of some of the privacy-protective tools and investigative methods they’ve developed.
The policy debates about AI are coming to a theater near you
In late April, the Federal Trade Commission along with three other federal agencies issued a joint statement emphasizing their collective commitment to leverage their existing legal authorities to protect the American people from AI-related unlawful bias, unlawful discrimination, and other harms. The statement identified that “Data and Datasets”, “Model Opacity and Access”, and “Design and Use” can all lead to potential discrimination in automated systems - and many of the laws that those agencies oversee apply to local and state data systems.
On May 23, USEd released a new report, “Artificial Intelligence (AI) and the Future of Teaching and Learning: Insights and Recommendations”. The White House announced the report–which it says “summariz[es]the risks and opportunities related to AI in teaching, learning, research, and assessment”–and a National AI R&D Strategic Plan, a roadmap that outlines key priorities and goals for federal investments in AI R&D. While the Strategic Plan focuses primarily on the federal government, many aspects of the report are equally relevant to state and local governments.
The White House also announced a Request for Information (RFI) seeking input on national priorities for mitigating AI risks, protecting individuals’ rights and safety, and harnessing AI to improve lives. In particular, data system implementers may want to weigh in on question 28: “What can state, Tribal, local, and territorial governments do to effectively and responsibly leverage AI to improve their public services, and what can the Federal Government do to support this work?” Other questions that may be of interest are 1-3, 9-10, 12-15, 17, and 19. Responses to the RFI are due by 5:00 pm ET on July 7, 2023.
The bottom line? Local and state data system staff should be aware of how existing laws are being interpreted to guard against AI harms and also watch for new laws and regulations.
Recommended resource: Does it feel like you don’t know where to start when it comes to AI adoption, data governance, and ethics? We recommend The Algorithmic Equity Toolkit’s fantastic–and short–questions about algorithmic impacts, effectiveness, and oversight.
It’s a good time to revisit your FERPA Knowledge
The White House announced that USEd “will promote and enhance the privacy of minor students’ data and address concerns about the monetization of that data by commercial entities, including by planning to commence a rulemaking under the Family Educational Rights and Privacy Act (FERPA).” What we’re not seeing so far is a nod towards the fact that FERPA doesn’t just regulate minor students: its protections and requirements extend to postsecondary students as well (and to the third parties receiving data under a FERPA exception). To prepare for FERPA fun, we recommend reading FERPA resources that are specific to data systems to see what might need a regulatory or legislative fix.
New Resources
USEd released two new guidance documents about FERPA, with a particular focus on student health records: “Family Educational Rights and Privacy Act: Guidance for School Officials on Student Health Records” and “Know Your Rights: FERPA Protections for Student Health Records.”
Data Quality Campaign (DQC) published “What Now? A Vision to Transform State Data Systems.” The resource and accompanying infographic explore what access people need to the information in data systems so they can make informed decisions and better navigate transitions.
ICYMI: Actionable Intelligence for Social Policy (AISP) published “Yes, No, Maybe? Legal & Ethical Considerations for Informed Consent in Data Sharing and Integration.” The resource explores the legal, ethical, and practical challenges of consent in data sharing and integration.